讲座编号:jz-yjsb-2013-y033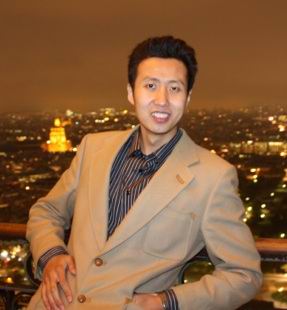
讲座问题:The Economics of Resource Sharing in Cloud Computing
主 讲 人:牛笛,加拿大阿尔伯塔大学(University of Alberta)
讲座时间:2013年05月15日(周三)下昼14:00
讲座所在:耕作楼809聚会室
加入工具:盘算机与信息工程学院西席、研究生
主理单位:研究生部
承办单位:盘算机与信息工程学院
主讲人简介:
牛笛博士自2012年9月起在加拿大阿尔伯塔大学(University of Alberta)---电子与盘算机工程系担当助理教授(Tenure-Track Assistant Professor)及博士生导师。他在多伦多大学(University of Toronto)取得了博士学位,师从盘算机网络通讯领域着名的李葆春教授(Dr. Baochun Li),在读时代获得了加拿大政府揭晓的“亚历山大贝尔”研究生最高奖学金和中国“国家优异自费留学生奖学金”。从2006年起,他在国际一流学术聚会和期刊上揭晓了20篇高质量文章,并且担当数十个顶级期刊和聚会的审稿事情。牛教授现为国际电气电子工程师协会(IEEE)和美国盘算机协会(ACM)的成员,与悠视网(UUSee), 微软研究院(Microsoft Research Redmond) 等海内外中大型企业有相助研究项目。
主讲内容:
Two economic issues are related to resource sharing : 1) How can the cloud reduce its operational cost through resource multiplexing while providing performance guarantees in face of varying demands? 2) How should the cloud competitively and fairly price its guaranteed services in the presence of resource sharing?
To answer these questions, I will present two case studies. First, we discuss cloud network reservation and pricing for a large number of QoS-sensitive clients. We formulate the problem as network utility maximization (NUM) with a coupled objective function, and propose a new distributed solution that proves to be more efficient and scalable than the traditional method of dual decomposition in practice. We also show that the optimal prices form a Nash Equilibrium and depend not only on the usage but also on workload patterns. In the second case study, we propose a cloud brokerage service that books a large pool of reserved instances from IaaS clouds and serves clients with price discounts. The broker exploits both the pricing gap of reserved and on-demand instances and the gain from time-multiplexing partially used instance-hours. We propose strategies for the broker to make dynamic instance reservation decisions, which can efficiently handle a large volume of client demand estimates. Our studies are verified by 400 GB of traces collected from a commercial video delivery service called UUSee and 180 GB of Google cluster usage traces.
泉源:研究生部 | 作者:|
宣布时间:2013-05-13 |
浏览量: